CEHC Professor Analyzes Economic Cost of Critical Infrastructure Disruptions
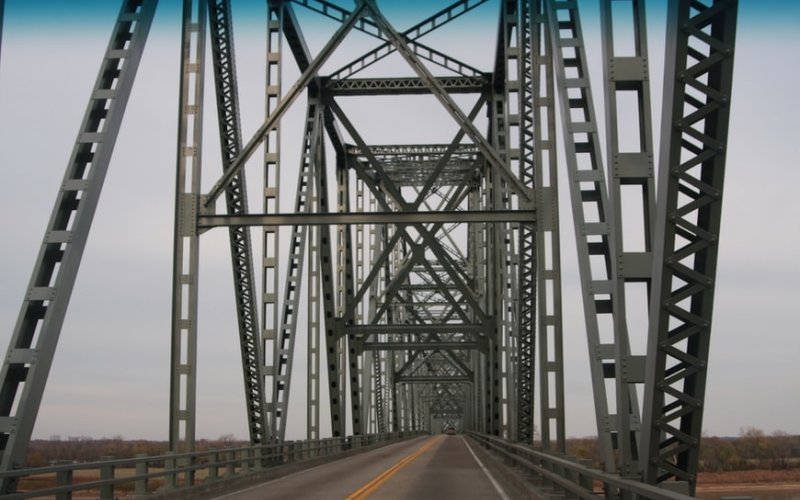
ALBANY, N.Y. (Dec. 16, 2021) – The resilience of America’s critical infrastructure networks has been tested over the last year by an increasing number of serious disasters – both natural and manmade.
For example, in February, a widespread power outage in Texas left more than 4.3 million people in the dark without heat (some for several days) as state leaders scrambled to fix the electrical grid failures. While notable cyberattacks, including a ransomware attack on the Colonial Pipeline in May and another on JBS USA Holdings, Inc., one of the world’s largest meat producers, just a few weeks later, had a disastrous impact on many sectors of the U.S. economy.
An analysis published by Unal Tatar of the College of Emergency Preparedness, Homeland Security and Cybersecurity (CEHC) and his colleagues is sharing new insight on the potential economic consequences of these infrastructure failures.
The group developed a new analytical model that simulates economic losses caused by disruptions of varying lengths and severity on the 10 most impacted critical infrastructure sectors: state and local general government; miscellaneous professional, scientific, and technical services; administrative and support services; federal government (nondefense); management of companies and enterprises; wholesale trade; computer systems design and related services; other real estate; insurance carriers and related activities; construction.
Their study, published in Risk Analysis, uses the model on a highway transportation network in Virginia. It indicates that economic losses experienced in just a single day from disruptions could range from approximately $8 million to $250 million, depending on the length and severity of the incident.
“The functionality of critical infrastructures, such as transportation, energy, and supply chains, is vital for health, safety, security and economic activity,” said Tatar, an assistant professor at CEHC. “While risk-informed physical network models and economic models have been well-studied, there is limited study of how physical features of network performance interact with sector-specific economic performance, particularly as these networks recover from disruptions of varying durations.”
Highway Infrastructure Disruptions
The Virginia highway scenario relied on publicly available daily traffic data in the Hampton Roads region. It includes 16 cities and counties, such as Virginia Beach, Norfolk and Newport News. The region’s metropolitan area accounted for more than $100 billion in GDP as of 2018, according to the study.
To test their approach – which provides a framework to integrate a Functional Dependency Network Analysis (FDNA) with Dynamic Inoperability Input-Output Models (DIIM) to calculate economic losses based on disruptions – the researchers considered three different scenarios:
- Mild, relatively frequent, conditions (a three-hour disruption)
- Moderately severe conditions (a six-hour disruption)
- Severe, infrequent conditions – such as evacuation during and after a hurricane (one- or two-day disruption)
Each scenario created a different level of inoperability for “nodes” in the highway’s transportation network (including bridges, tunnels, specific sections of the highway). That inoperability ranged from about 15 percent for a mild disruption to 85 percent after a hurricane evacuation.
The severe scenario resulted in economic losses as high as $256.42 million in a single day, while the mild scenario resulted in $8.07 million in losses and the moderate scenario resulted in $12.49 million in losses.
“Disruptions on single or few nodes can have a drastic impact on network functionality,” Tatar said. “The type of modeling presented in this paper can help critical infrastructure managers who seek to prioritize investments in network reinforcements activity. Moreover, calculating the economic impact of disruptive scenarios will improve risk communication for the public.”
Tatar’s co-authors included Joost Santos of George Washington University and Shital Thekdi of the University of Richmond.
Their findings were presented at this month’s Society for Risk Analysis Virtual Annual Meeting.