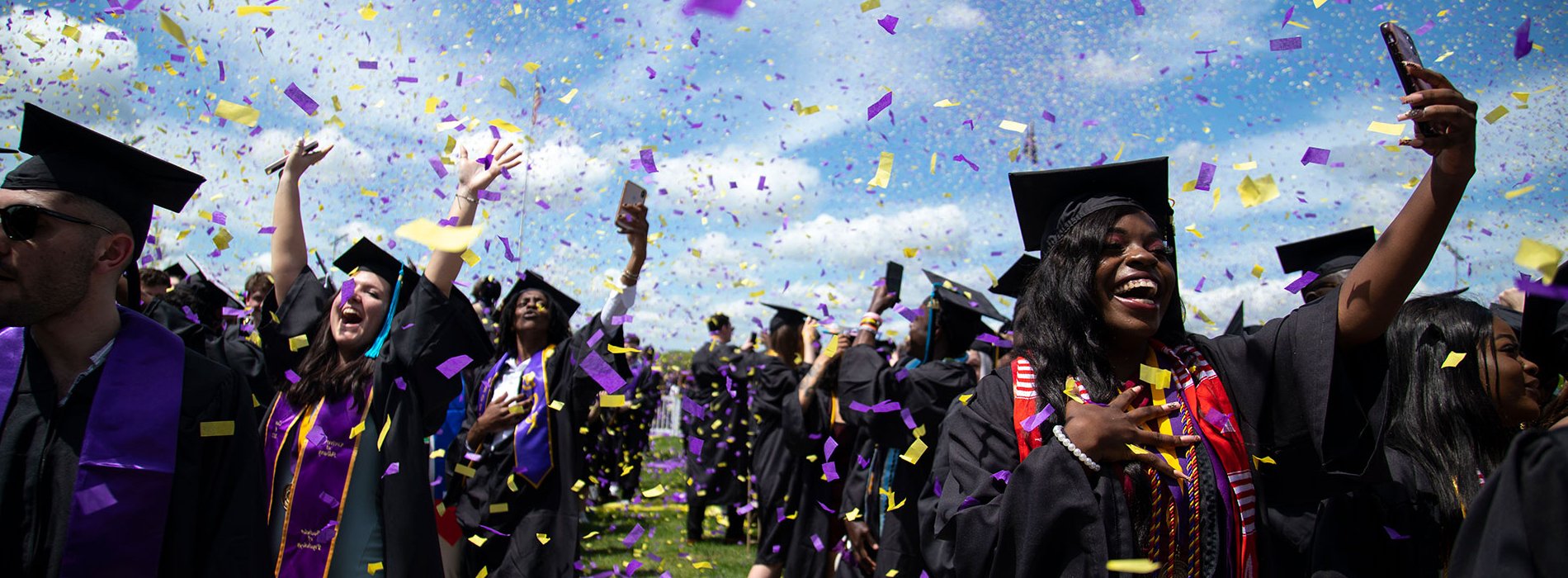
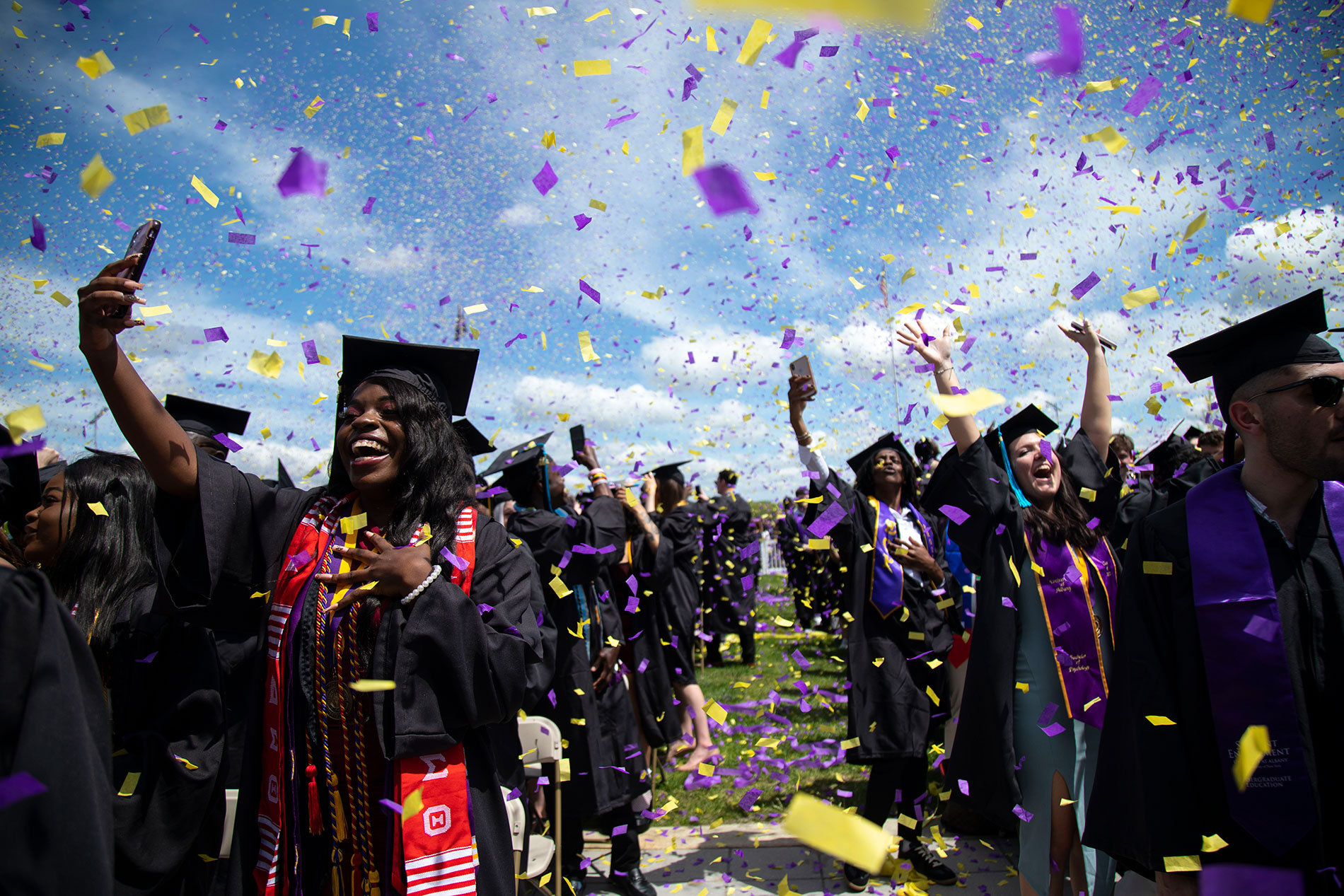
Great Danes Rising: Unleashing Our Potential
The University at Albany Strategic Plan 2024-2029
Welcome to Great Danes Rising: Unleashing Our Potential, our University's exciting new strategic plan. Building upon the remarkable achievements of our prior plan, Authoring Our Success, we are poised to embark on a transformative journey that will propel us even further toward excellence.
The University at Albany is, thanks to our faculty, staff, students and alumni, experiencing great momentum in all five of our core priorities: student success, research excellence, diversity and inclusion, internationalization, and engagement and service. These priorities will guide our path to success.
The five core priorities of our Strategic Plan remain intact from the prior plan, as they collectively express UAlbany’s unique assets—and our aspirations for the future. They are the touchstones that will anchor the next chapters in our already long and rich history.
We support these five priorities with three main strategies, advanced through institutional initiatives that will be measured through data points, such as retention, increased funding, engagement, participation and enrollment. In this Strategic Plan, we move forward with concrete plans, many based on ideas we have already begun to implement.
As we embark on this ambitious journey, we invite all members of our University community to join us in unleashing our full potential. Together, we will rise to new heights of achievement, impact lives, and shape a brighter future for all, and with your help and input, we will power the University at Albany’s momentum, research and rising reputation.
This is our time to unleash the great potential of the University at Albany.
The University at Albany is an engine of opportunity. Fueled by our unique mix of academic excellence, internationally recognized research, and world-class faculty, we relentlessly pursue possibilities, create connections, and open opportunities—locally and globally—with a single-minded purpose: To empower our students, faculty, and campus communities to author their own success. This is the University at Albany.
To be the nation’s leading diverse public research university—providing the leaders, the knowledge, and the innovations to create a better world.
ACCESS
To enable individuals to pursue learning, research, and service regardless of economic, societal, or physical factors.
INTEGRITY
To be committed to—and expect from all—honesty, transparency, and accountability.
INCLUSIVE EXCELLENCE
To value diversity of all forms, academic freedom, and the rights, dignity, and perspectives of all individuals.
COMMON GOOD
To work collectively and collaboratively to benefit our communities—and create a sustainable way of life on earth.
Student Success | Research Excellence | Diversity and Inclusion | Internationalization | Engagement and Service
We will be the nation’s leading student-ready research university, empowering our diverse student body for a lifetime of achievement.
Strategy 1: Maximize students’ personal and intellectual growth while fostering their health, well-being and engagement.
One way we’ll get there: “Just for the Health of It” – A campaign to promote student well-being and build upon our designation as a health-promoting university.
We will measure success by increases in the retention rate and measures of belonging in NSSE and other UAlbany survey results.
Strategy 2: Create individualized academic pathways that ensure all students have a roadmap to a timely graduation.
One way we’ll get there: Introduce “Finish in Four” – For all new Full Time First Time undergraduate students, a campaign with advising and other student support centers to help students finish their degree on time.
We will measure success by improving the six-year graduation rate and closing retention gaps and perceptions of health/wellbeing for students across race/ethnicity demographics.
Strategy 3: Position every student for career success through experiential learning opportunities including research, internships, service learning and education abroad.
One way we’ll get there: “UAlbanyXEd for all” – Experiential education (XEd) opportunities available and accessible to all students.
We will measure success by increasing the student participation in experiential and applied learning opportunities and employment or graduate school attendance post-graduation.
Tamia Wellington’s path to UAlbany was a non-traditional one, but once she got here, she embraced fully her life as a Great Dane: a busy biology major with minors in creative writing, psychology, and educational studies, Tamia was proud of her work as an undergraduate research assistant and her leadership role as president of the Middle Earth peer assistance program. From studying the impact of toxins on human and environmental health to helping fellow students navigate challenging times, she found a synergy for her future career goals in the medical field. “It's really important to be able to hear your patients and what they're saying, and understand them. I never want to be in a position where my patients feel as if they're not being heard or understood, because everyone has a story,” she says.
We will be internationally known for our research and creative works impacting critical societal challenges.
Strategy 1: Strengthen interdisciplinary research networks and collaborations across the academic enterprise.
One way we’ll get there: Uniting Frontier Research and Development – Following the successful creation of the Institute for Social and Health Equity and the upcoming establishment of the Institute for Artificial Intelligence, we will continue to institutionalize our research strengths by fostering research communities to address grand societal challenges, with initiatives such as the Healthy Aging Initiative and the Global Center for AI in Mental Health.
We will measure success by increasing the number of large-scale interdisciplinary research proposals submitted from 1-2 to 10 each year and increasing the percentage of research-active faculty.
Strategy 2: Grow research and scholarship in all disciplines by leveraging our investments in research infrastructure
One way we’ll get there: Capital funding – Requests to federal/state government in areas with national importance, such as nanotechnology, semiconductor development and artificial intelligence, for additional funding.
We will measure success by an increase in the total research portfolio and the number of externally funded organized research proposal submissions.
Strategy 3: Collaborate with partners in industry and government to drive economic and workforce development.
One way we’ll get there: UA-CREATE – UAlbany’s signature innovation and entrepreneurship ecosystem.
We will measure success by an increase in the number of technology disclosures, patent applications, and licensing and faculty-associated startup companies, as well as an increase in the number of postdocs and graduate students who are funded by industry and government.
Nanoscale science major Julia Garrison is exploring new pathways in nanotechnology and biochemistry in an effort to develop new and innovative approaches to curing cancer. In her photochemistry lab, she’s researching how to use lithography techniques for biological applications using light-sensitive chemicals – ultimately to develop model organs of the human body. The goal of the research is to allow tissue engineers the ability to grow tissues and organs that cannot be made with existing solutions. “Personally, I'm interested in the salivary glands because treatment for that is not very advanced right now,” she says. “It's hard to treat salivary gland cancers. I want to be able to help people who have these types of cancers as well as cancers that we might not know that much about.”
We will be the preeminent model for embracing diversity, equity, and inclusive excellence in all facets of university life.
Strategy 1: Diversify the University’s workforce through evidence-based recruitment and retention practices.
One way we’ll get there: Linking to SUNY’s Diversity, Equity and Inclusion priorities, including strong participation in the leadership institutes, PRODiG, and the DEISJ Fellows program.
We will measure success through a more diverse applicant pool and increasing the number of faculty and staff from historically underrepresented groups.
Strategy 2: Expand the breadth of diverse perspectives in our curricular, co-curricular and workplace experiences.
One way we’ll get there: Reclaiming History and Renaming Spaces – The designation of various campus spaces and facilities to highlight historical figures who may have been marginalized or ignored by traditional history texts, or to celebrate UAlbany alumni who, over the past 180 years, have changed society for the better.
Examples include Parker Pond, named for three indigenous students who attended the predecessor of the University at Albany in 1844, and Harvey House, an intentional community within the Dutch Quad complex that supports and affirms the cultural experiences of queer and transgender students, is named for Harvey Milk, class of 1951, a politician and activist who became one of the first openly gay elected officials in the nation.
We will measure success through measures of inclusivity in a climate survey.
Strategy 3: Advance our national reputation for creating social mobility for first-generation and historically underrepresented students—and for empowering a new generation of allies, leaders and changemakers.
One way we’ll get there: The creation of a social justice curriculum – An interdisciplinary minor that prepares students to engage in personal change, dismantle inequitable systems, and advocate for a just society.
We will measure success through recognitions, certifications and grant opportunities.
When Almas Abbas took chemistry in high school, she faced a learning environment challenged by COVID restrictions, which she felt left her unprepared. At UAlbany, she found support at the Center of Achievement, Retention and Student Success (CARSS), which offers academic assistance to students enrolled in science courses and is made possible in part by the HHMI Driving Change initiative. This initiative works to ensure undergraduate students from all backgrounds, particularly those who belong to historically excluded groups, will excel in STEM and graduate from college well-prepared to pursue advanced degrees and assume leadership roles in STEM fields. “CARSS definitely does encourage diversity and inclusion,” she says. “You see a bunch of people from different cultures and you also see others trying to understand these different cultures in CARRS tutoring sessions. Understanding different mindsets can help you grow, gain knowledge, be successful and gain friendships.”
We will infuse international perspectives across our research, teaching, learning and service to promote the global common good.
Strategy 1: Deliver intercultural and international learning opportunities across the disciplines.
One way we’ll get there: UAlbany Go Global – An integrated campaign of four actions: strengthening advisement capabilities, curricular integration, scholarship and University-wide support, by providing additional support for navigating and financing the transformative but complex study abroad experience.
We’ll measure success by an increase in student participation, course offerings available abroad and faculty engagement.
Strategy 2: Expand international partnerships that strengthen research, increase enrollment, and provide new opportunities for students, faculty, and alumni.
One way we’ll get there: UAlbany Global Handshake – International enrollment and productive international partnerships will be incentivized through strategic investments designed both to leverage recruitment and partnerships in which UAlbany has demonstrated strength, and in new opportunities to develop recruitment and institutional engagements in parts of the world where the University’s global footprint is less prominent.
We’ll measure success by an increase in the number of productive international partnerships.
Strategy 3: Recruit and retain students and faculty from a broader range of countries.
One way we’ll get there: UAlbany Calls! – The University will promote the growth of recruitment of international students and visiting faculty from underrepresented countries through strategic investments (see initiative for Strategy 2) and the active promotion of funded opportunities for visiting international scholars, working with colleges, schools, departments, CIEGS and the Division for Research and Economic Development, to host international visiting scholars from underrepresented countries.
We’ll measure success by doubling the number of students and international students from underrepresented geographic areas globally who attend UAlbany.
MPH student Aicha Akindele, whose family lives in the West African nation of Togo, spent her summer interning abroad with the Togolese branch of the United Nations Population Fund (UNFPA). While in Togo she supported efforts to improve sexual and reproductive health, gender equality, and population data, and she plans to combine what she learned abroad with her academic coursework to prepare for a career in global maternal and child health. Interning abroad, she says, “strengthened my passion for global health and solidified my commitment to working toward improving maternal and child health outcomes in underserved communities.”
We will build transformational partnerships that strengthen the University and our local and global communities.
Strategy 1: Create an institutional culture that values and measures mutually beneficial partnerships with our communities.
One way we’ll get there: Re-establish the role of the Provost Fellow for Publicly Engaged Research and Teaching within the Office of Academic Affairs to help foster a campus culture that recognizes and supports community-engaged and transdisciplinary research, teaching and learning.
We’ll measure success by increasing the number of faculty and staff who report engaged research, teaching and service and by doubling the number of students enrolled in a course with an attribute of public engagement, community service or related descriptors.
Strategy 2: Respond to our communities' needs through faculty, staff and student knowledge and engagement, and by leveraging University facilities and assets.
One way we’ll get there: Designate the Office of Government and Community Relations as the initial point of contact and event liaison for external groups and community partners who want to hold events on campus (e.g., Hugh O’Brien Youth Leadership, NYS Education Department Statewide First in Math Competition, Bloc Power, Dwyer Peer-to-Peer, Governor’s and state agency events, and Congressional events).
We’ll measure success by increases in publicly engaged teaching and scholarship.
Strategy 3: Increase community member participation in University events and programs.
One way we’ll get there: Open to the Community – A series of both new and expanded events that invite the community to explore what our campus has to offer. Events include Showcase, Research & Entrepreneurship Week, and the Big Event community service day, and new events such as a Community Partners Thank You for Capital Region organizations and agencies, a Community Block Party in connection with an athletic event, and NANOvember, public programming to highlight nanotechnology.
We’ll measure success by increases in public attendance at events and a community survey of satisfaction.
Department of Atmospheric and Environmental Sciences Sr. Research Support Specialist Nick Bassil believes that community-engaged research is vital to a university’s research enterprise because its outcomes directly impact people’s lives and livelihoods. Public engagement, he says, is all about “connecting the dots for people who know the starting dot and the last dot” of a problem, but who need help figuring out how to navigate the journey in between. In his work with utility companies across New York State to improve their ability to forecast weather and outages, that means developing solutions to help people solve problems, improve their businesses, save money – and even lives.
In spring 2022, Carol Kim and Mike Christakis, as Council co-chairs, hosted 11 forums which welcomed nearly 450 faculty, staff and students across UAlbany’s schools, colleges, administrative divisions, and shared governance groups.
Current members include:
Strategic Plan Advisory Council
Carol Kim, Provost and Senior Vice President for Academic Affairs, Co-Chair
Michael Christakis, Vice President for Student Affairs, Co-Chair
Student Success
JoAnne Malatesta, Dean of Undergraduate Education and Vice Provost for Academic Innovation & Student Success, Co-Chair
Ed Engelbride, Associate Vice President for Student Affairs, Co-Chair
Vanessa Borum, Associate Director for Academic Advising
Kristen Swaney, Assistant Vice Provost for Undergraduate Education, Director of Academic Advising
Billie Franchini, Director of the Center for the Advancement of Teaching, Learning & Online Education, Interim Director of Online Learning, University Senate Rep
Doug Sweet, Director, Student Affairs Assessment and Planning
Research Excellence
Kevin Williams, Interim Dean of the Massry School of Business, Co-Chair
Kesh Kesavadas, Vice President for Research & Economic Development, Co-Chair
Andy Berglund, Director of The RNA Institute, Professor - Department of Biological Sciences
Theresa Pardo, Associate Vice President for Research & Economic Development
Jen Goodall, Vice Dean, College of Emergency Preparedness, Homeland Security and Cybersecurity, University Senate Rep
Steven Doellefeld, Director of Assessment
Diversity & Inclusion
Jeanette Altarriba, Dean of the College of Arts & Sciences, Co-Chair
Samuel Caldwell, Associate Vice President & Chief Diversity Officer, Co-Chair
Christine Wagner, Interim Vice Provost and Dean of the Graduate School
Julia Hormes, Associate Professor, Department of Psychology
Sean Rafferty, Professor, Department of Anthropology, University Senate Rep
Internationalization
Gilbert Valverde, Vice Provost & Dean, International Education & Global Strategy, Co-Chair
Rita Biswas, Associate Professor of Finance, Massry School of Business
John Justino, Clinical Associate Professor, School of Public Health
Timothy Sergay, Associate Professor of Russian, Department of Languages, Literatures & Cultures, University Senate Rep
Emily Feuer, Chief of Staff, Division of Finance and Administration
Engagement & Service
Victoria Rizzo, Interim Dean of the School of Social Welfare, Co-Chair
Sheila Seery, Vice President for Government & Community Relations, Co-Chair
Mary Hunt, Director of Public Engagement
Janine Jurkowski, Department Chair and Professor, Department of Health Policy, Management and Behavior
Sheri Stevens, Director, Community and Public Service Program
George Berg, Associate Professor, College of Emergency Preparedness, Homeland Security and Cybersecurity, University Senate Rep